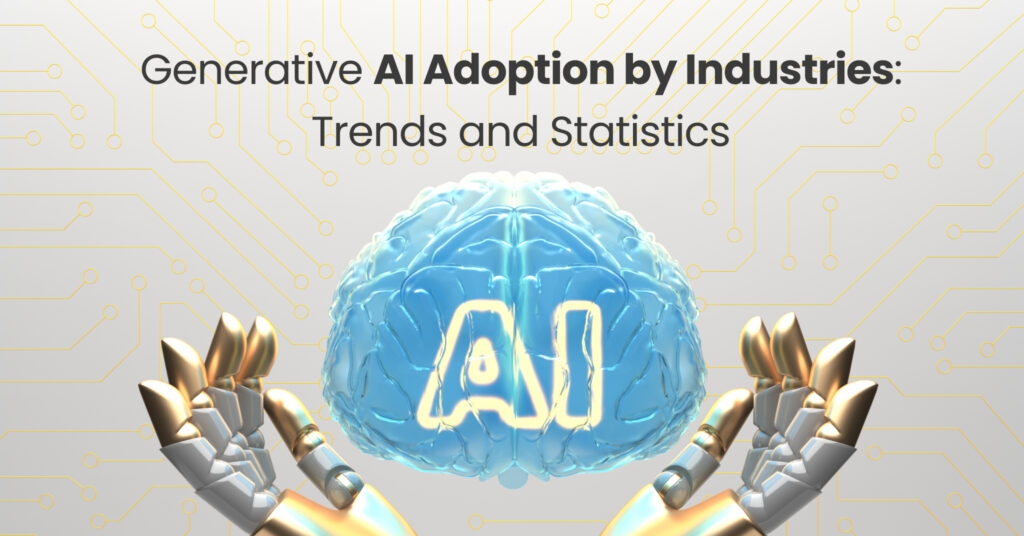
In recent years, generative AI has seen an exponential rise in adoption across industries. Powered by advanced algorithms, vast datasets, and increased computing power, its creative potential is being leveraged for content generation, user personalization, and innovative research. This widespread adoption highlights generative AI’s transformative role and the growing trend of human-AI collaboration.
The market size of AI is expected to grow at a compound rate of 46.47% [Source: Statista] annually for the next six years, contributing up to $15.7 trillion [Source: PwC] to the global economy by 2030. Generative AI-the kind that is creative and collaborative-constitutes a bulk of the size.
Generative AI has come a long way, from creating simple chats to generating lengthy, grammatically sound text, like the opening paragraph here—which is entirely and as it is generated with AI. It also has a long way to go. This juncture, therefore, is an apposite vantage point for us to consider the impacts, trends, and challenges associated with generative AI.
Impact of Generative AI on the Economy
Most business leaders (74%) consider generative AI a top emerging technology that will impact their business in 2024. Generative AI will take over most redundant and menial tasks and allow employees to focus on core and critical parts of work.
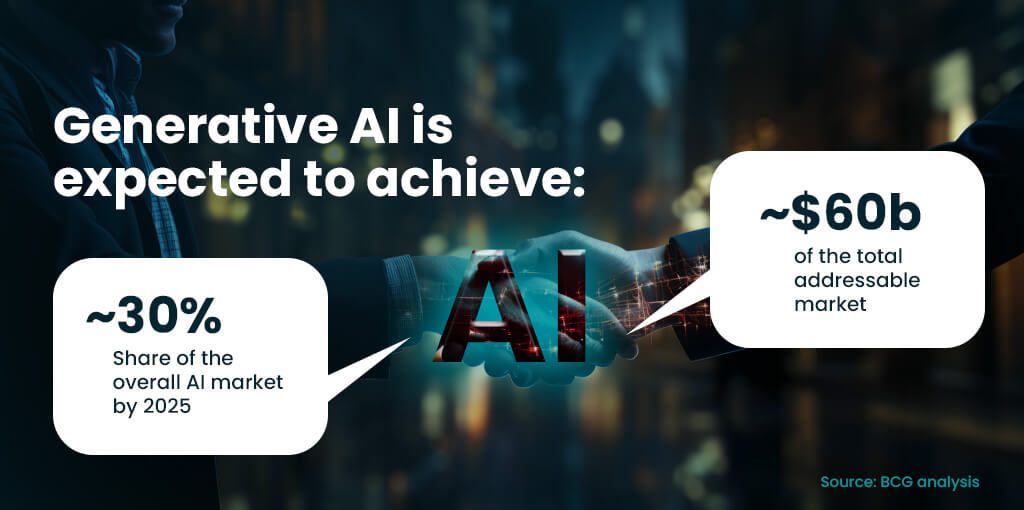
Technologies related to generative AI will increase productivity and economic output. A recent EY report suggests that adoption of Generative AI in enterprises has significantly increased (around 30-40%) within the first few months of 2024. The technology is quickly being adopted for internal employee-facing processes. Approximately one-third of the use cases involve using smart assistants for performing specific tasks, while another 25% pertain to marketing automation leveraging various tools.
Goldman Sachs further supports these claims, estimating that task automation through generative AI could add almost $7 trillion to the global GDP and increase productivity growth by 1.5 percentage points over a decade.
Generative AI will have a significant impact on most industries—mostly positively. Industry experts suggest that, by 2030, around 30 percent of jobs could be automated thanks to generative AI. Jobs most exposed to this include office support and production work, where 87% and 82% of tasks, respectively, could be potentially automated. The Microsoft Work Trend Index data reveals that 70% of early Copilot users have experienced increased productivity due to task automation. Additionally, 68% report that Copilot has enhanced the quality of their work.
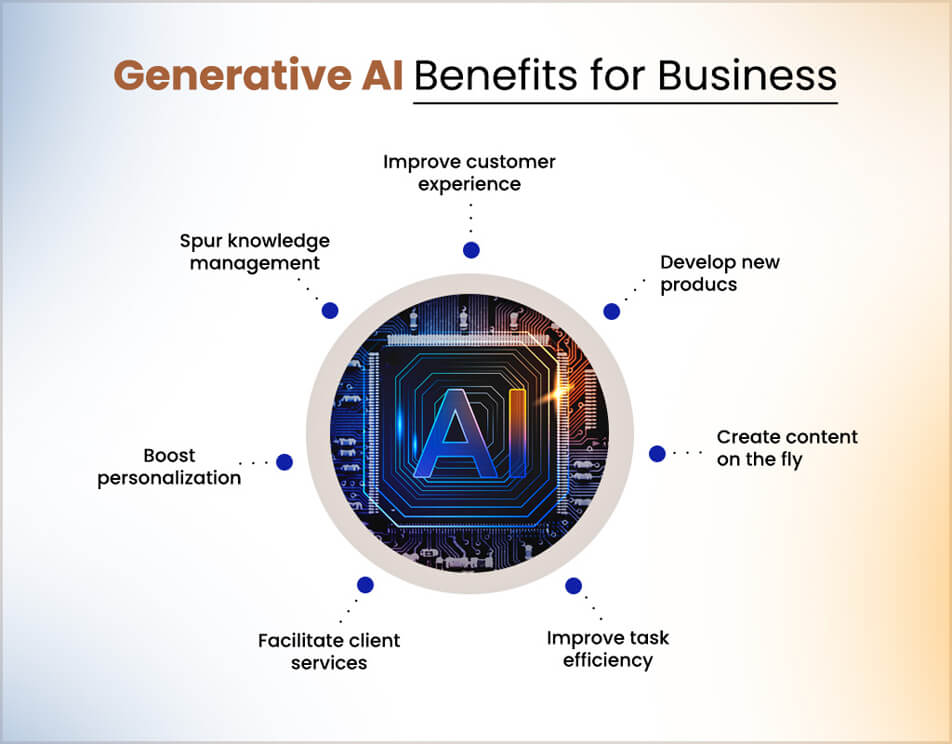
However, automation will not render humans superfluous. Instead, it might lead to the transformation of job roles, increased efficiency, and the creation of new opportunities. For instance, while high-end generative AI tools like Claude and Hypotenuse AI have created doubts about the profitability of hiring writers, AI-content editing has gained significant attention from relevant domains.
According to the Oliver Wyman Forum’s analysis, generative AI and automation are likely to impact three types of tasks: transactional, relational, and expertise-related. Transactional and relational tasks are expected to be automated through a mix of robotic process automation, machine learning, and generative AI, while expertise-related tasks will still necessitate human collaboration.
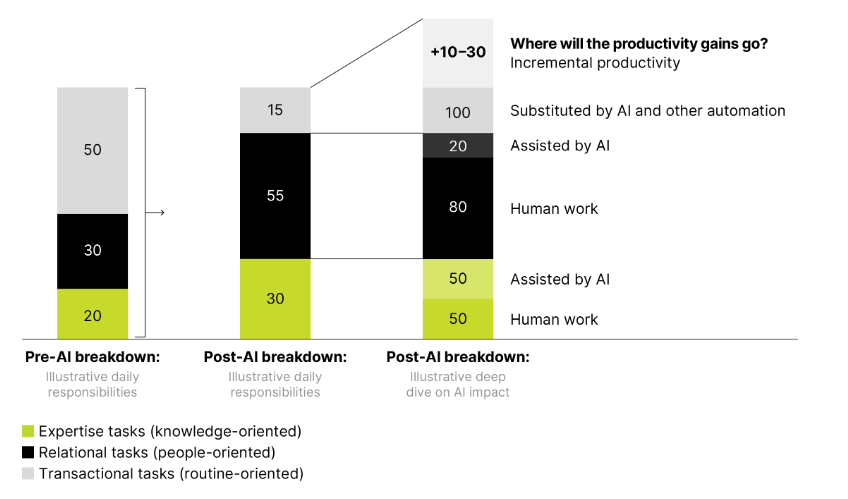
The impact of generative AI on the quality of jobs is of greater significance than the quantitative impacts. A survey by Upwork reveals that a majority of C-suite executives said their organizations will hire more professionals of all kinds because of generative AI—this is so that they can adapt to the technology and also deal with new tasks that will arise as a result. This should allay fears that generative AI will put humans out of work.
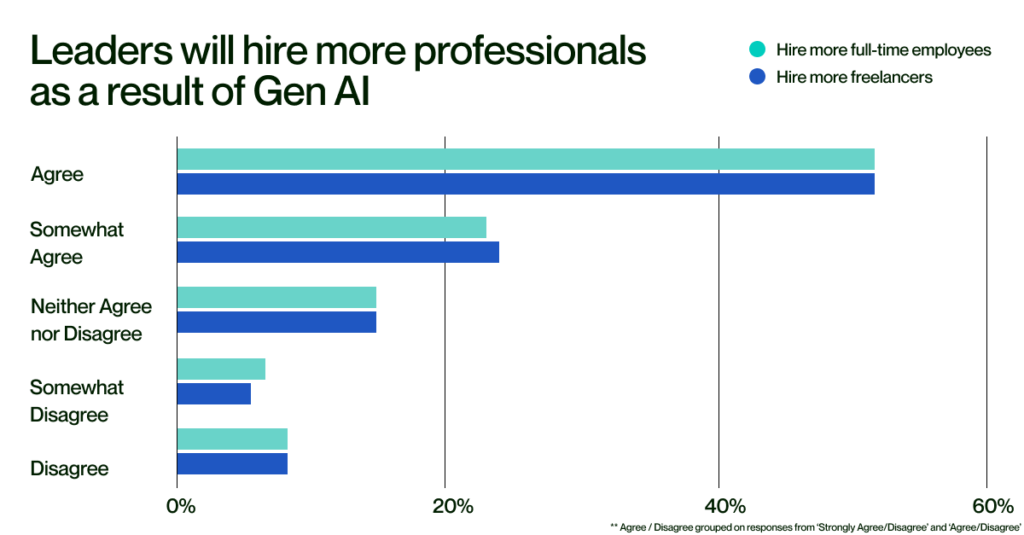
Protect your brand from the risks of AI-generated text.
Get expert human editors to enhance AI-written content.
Trends in Generative AI Adoption
With the numerous benefits it offers, generative AI continues to see widespread adoption across diverse industries. The McKinsey survey highlights a significant jump in adoption rates, from 33% in 2023 to 65% in 2024. Moreover, 50% of surveyed organizations report implementing AI across at least two business functions.
- Marketing and sales are the functions where generative AI is most widely used, with 34 percent of respondents saying their organization uses it for tasks associated with them. This is not surprising because marketing is a field that involves lots of tasks that generative AI excels at.
- Product & service development and IT are two other functions for which it is most widely used.
- Generative AI penetration is lowest in manufacturing, supply chain management, and HR. Only four percent of C-suite executives say their organization uses generative AI for manufacturing-related tasks.
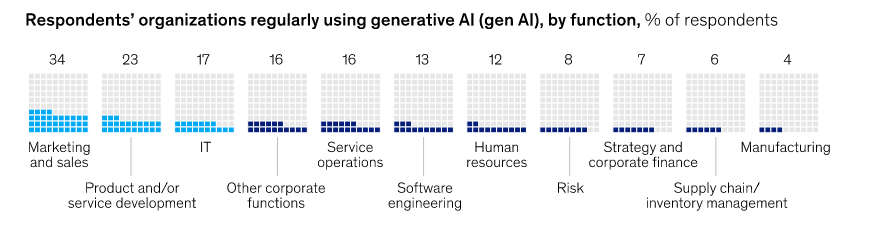
Gartner estimates that about 30 percent of outbound marketing content will be generated using AI by 2025.
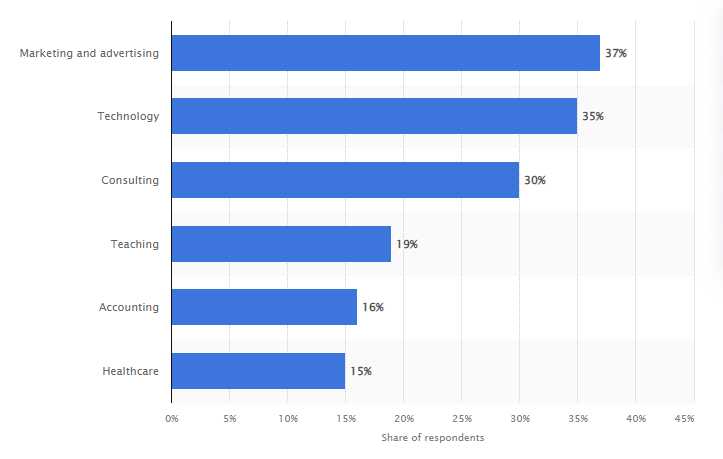
Software engineering, though, is the field with the most share of skills augmentable by generative AI. According to the LinkedIn report, only three percent of software engineering tasks need specialized human skills.
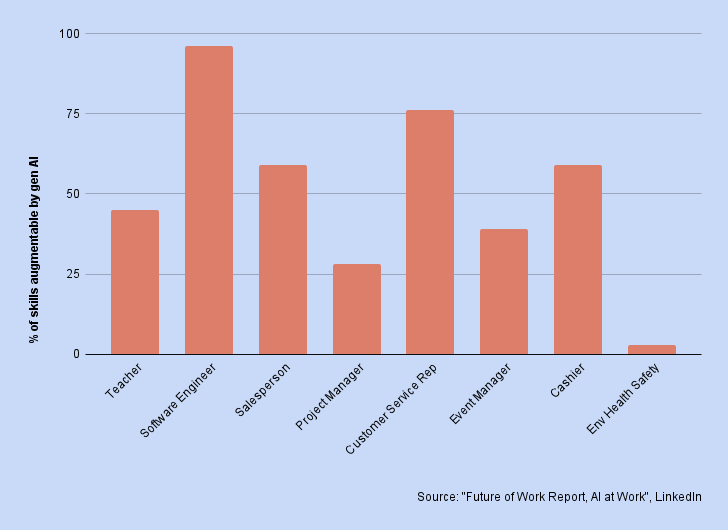
The same McKinsey survey also highlights that the adoption of generative AI results in a reduction in cost and an increase in revenue. The impact is most pronounced in HR operations, where 50 percent of respondents say their organizations saw a decrease in cost, and 33 percent, an increase in revenue, as a result of adopting generative AI.
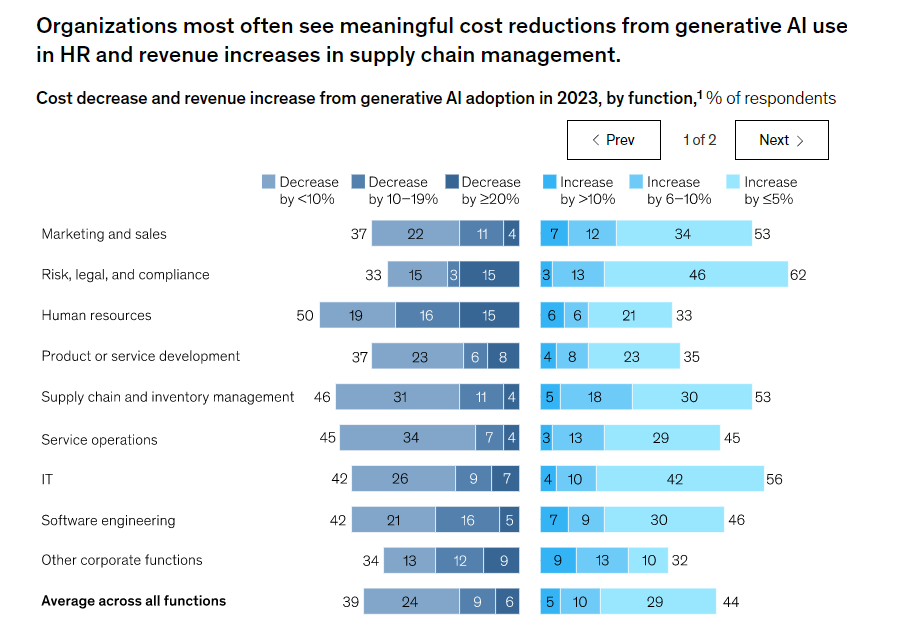
Human resources is just one example that benefits from incorporating generative AI in its process. Enterprises could use generative AI to deliver operational efficiency, enhance product offerings, offer new experiences, or enforce operational changes.
Interest in generative AI has therefore soared and so has investment in AI-related technologies. Goldman Sachs projects that investment in AI could reach up to 4 percent of GDP in the US in the next 10 years, with the generative kind taking up a major share. An exclusive KPMG survey highlights that around 77% of chief executives across industries consider generative AI the most influential emerging technology. Moreover, 71% of these executives plan to deploy generative AI solutions within their organizations over the next two years.
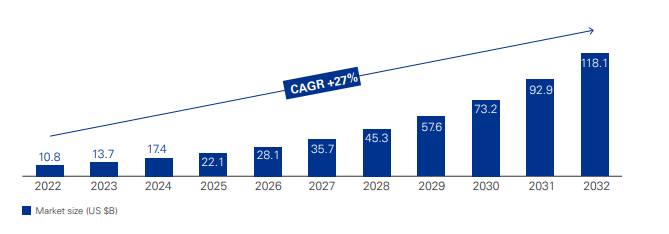
The increase in investment and the wider adoption of generative AI will lead to a fundamental shift in the nature of work and business operations. They will have a significant impact on research and development, product development, and business operations. The result of all this is higher innovation, increased efficiency, and higher customer satisfaction; and of course, the flip side: concerns with inaccuracy, bias, data privacy, and losing human connection, among others.
How to Scale Content Creation with AI (and Why Human Intervention is Still Required)
Challenges, Concerns, and Prospects of Generative AI
Generative AI has delivered much and still promises plenty. The challenges and concerns that it is fraught with are in nearly equal proportion. Some of these challenges are intractable, and some are even insoluble.
Substantial quantities of data are required to train and assess the generative AI models. However, without experienced data annotators, gathering, refining, and annotating data for model training can be tedious, costly, and difficult.
Generative AI models are only as good as, among others, the training data and computational resources—both of which are finite—and so they will always occasionally falter. Therefore, though organizations are keen to adopt generative AI, they also remain wary of it.
According to Statista, the majority of organizations (56%) consider inaccuracy in outcomes as a relevant risk with generative AI. Cybersecurity is the second major concern: 53% of respondents consider it a risk. Intellectual property rights violations and regulatory compliance are the next two. Workforce/labor displacement is another associated risk. 34% of organizations regard it as a risk with 13% working toward mitigating it.
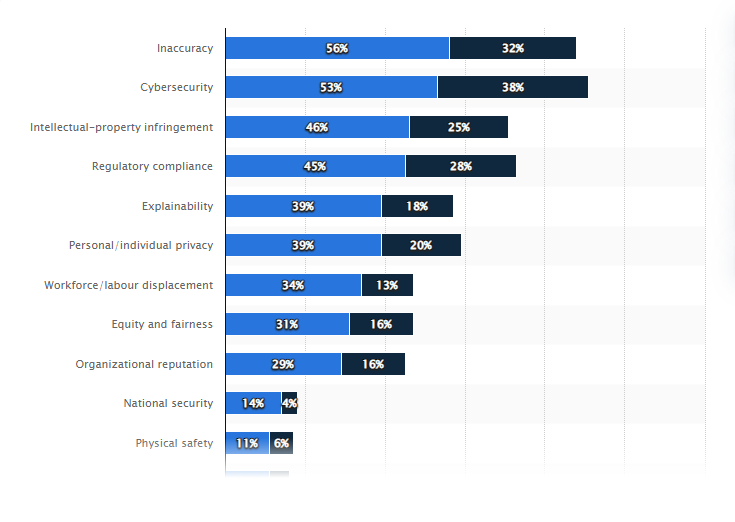
Generative AI may not necessarily render humans jobless, but it necessitates them to reskill and re-orient their strategy. Human traits such as analytical judgment, flexibility, and emotional intelligence are essential to counterbalance generative AI.
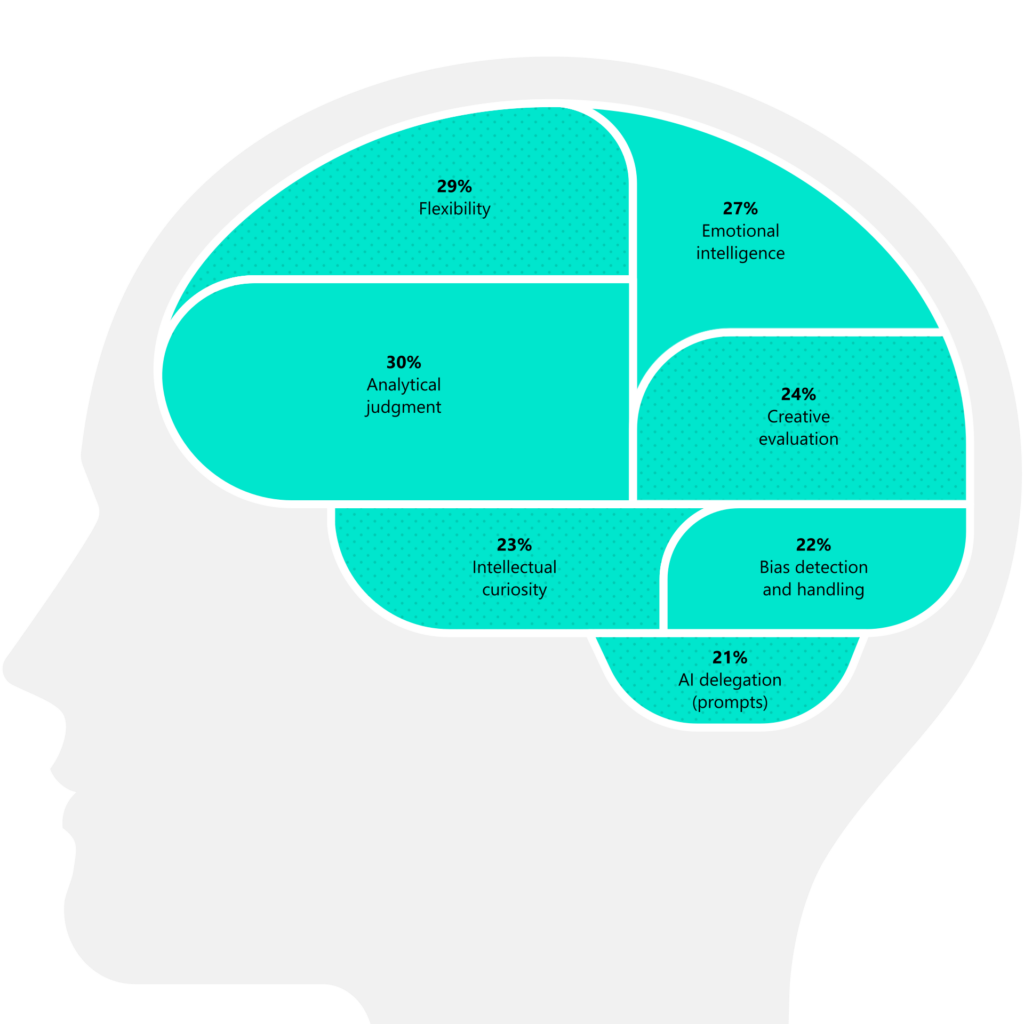
There is a gendered aspect to this, too. Women are two times more likely to be affected by automation—since the types of work they generally do are also those most susceptible to AI—as a result of generative AI, according to the ILO. Organizations must not turn a blind eye to this. They need to ensure that efforts to mitigate job displacement by generative AI are undertaken in proportion to the degree of its impact on workers.
Want to make your AI system qualitatively impactful?
We make that possible with bespoke data labeling services
The Necessity for Humans in the Loop of Generative AI
Impressive as they are, generative AI is not completely failsafe, and not likely to be so anytime soon. It is constrained mostly by the availability of data. AI still requires human oversight and involvement to ensure its outputs are ethical, accurate, and aligned with intended goals.
The many ways generative AI models can go awry and their ramifications necessitate human involvement and oversight—not just during training (in the loop) but also verifying the output (outside the loop). Humans are needed to carefully curate the datasets used, prepare them for AI training through annotation, consider potential biases and gaps, set the objectives and constraints for generative models, test the system, flag inaccurate or inappropriate outputs, and provide additional feedback to enhance the system’s performance.
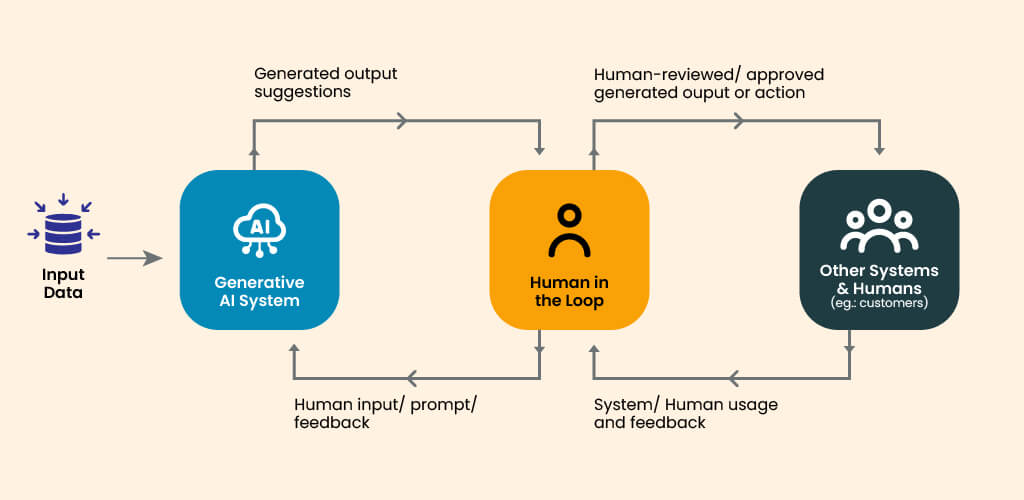
For instance, for high-stakes applications of AI, like digital revenue recovery software, ESG impact reporting platform, or smart parking applications, manual oversight by domain experts is essential to validate AI-generated insights and recommendations. Even for lower-risk applications like creating marketing copy or conversational bots, human reviewers can spot problematic outputs before public release.
Thus, a humans-in-the-loop approach is not just ideal when working with AI; it is pragmatic.
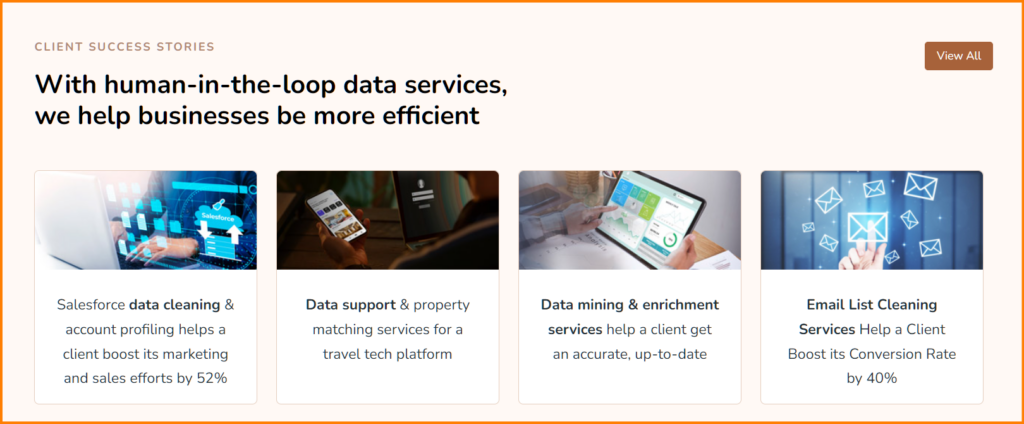
Endnote
As generative AI becomes more prominent in our lives, ensuring that the technology is accurate, fair, and safe is paramount. Human oversight cannot be overlooked. Doing so would mean letting AI systems run our lives with neither them nor us knowing what’s going on-inside them or outside.
Therefore, rather than full automation, responsible adoption of generative AI entails keeping humans firmly in the loop. By coupling human creativity and ethics with AI’s scalable capacity, generative systems can be safely harnessed to amplify human capabilities for years to come.