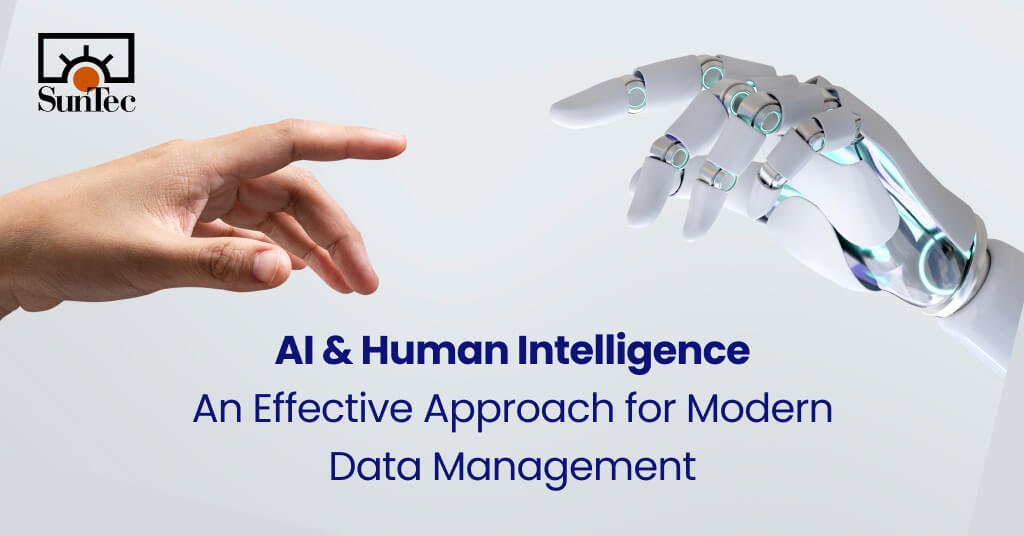
As businesses increasingly recognize data as their most valuable asset, the focus has shifted from just collecting information to managing it securely and efficiently. Modernizing data management processes has become a top priority, with organizations investing in advanced technologies such as AI and ML to address challenges like data governance, integration, and security. But here’s the catch— incorporating AI in data management alone isn’t enough. An efficient way of tackling data management challenges is combining AI with human expertise, creating a balanced approach that ensures data is used and managed responsibly. In this blog, we’ll explore how this synergy redefines the landscape of data management and why it’s essential for driving sustainable business growth.
Table of Contents
How Is AI Reshaping Data Management – Modern Approaches
The incorporation of AI in data management has been a transformative force, effectively addressing challenges across different stages of data lifecycle—from collection to storage and analysis. Artificial intelligence supports modern data management through:
Process Automation
Data professionals spend a significant amount of time and effort in processing and managing big data in real-time. McKinsey predicts that by 2030, up to 30% of global work hours could be automated. Leveraging RPA (robotic process automation), businesses can automate repetitive tasks such as data extraction, entry, and deduplication, significantly reducing processing time. Moreover, by integrating AI with RPA, companies can go a step further, enabling intelligent automation that can learn and adapt over time. This makes data management processes not only more efficient but also smarter.
Master Data Management (MDM)
To ensure uniformity, accuracy, and stewardship across the organization’s shared master data assets, businesses are increasingly focusing on a technology-enabled discipline known as MDM (master data management). It enables organizations to establish a single, reliable source of truth for critical data about products, customers, assets, and finances, helping to eliminate inconsistencies. A Markets and Markets survey projects the global MDM market will reach $34.5 billion by 2027, driven by the growing need for compliance and access to advanced data management tools.
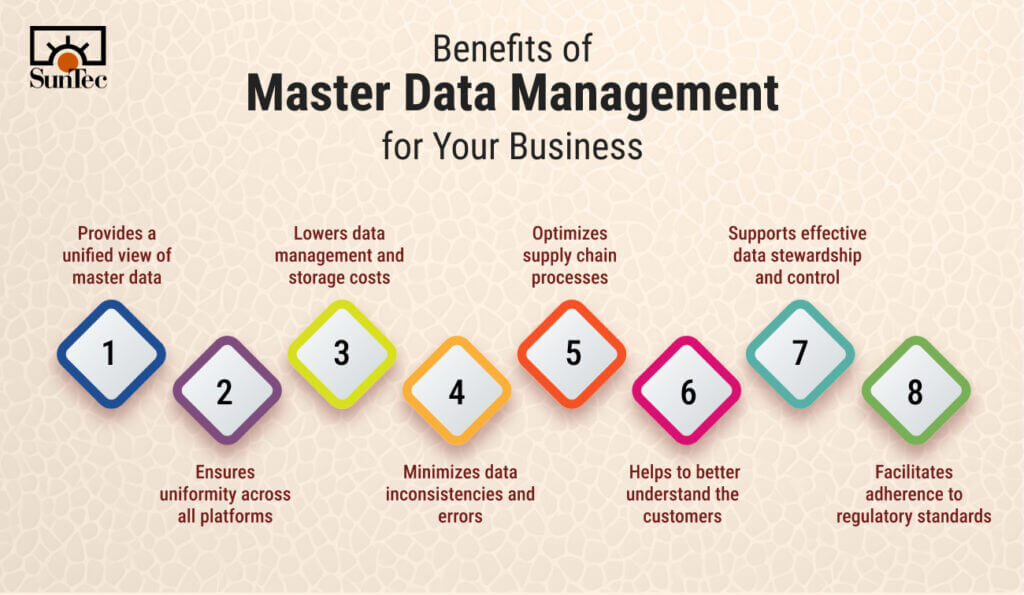
Artificial intelligence is streamlining the MDM process by automating ETL pipelines – including data extraction, standardization, mapping, and integration across systems. AI-powered tools can automatically detect and resolve data inconsistencies, such as duplicate entries or outdated information, ensuring that all departments rely on consistent and accurate data. The impact of this automation is profound—reducing errors, cutting down processing times, and enabling faster access to critical data for strategic initiatives.
Data Fabric
Another technology-centric data management approach that facilitates uniform distribution of data storage across multiple environments is data fabric. Rather than centralizing databases in data lakes, this metadata-driven approach integrates diverse data pipelines and cloud platforms using automated, intelligent systems.
Imagine AWS handling the critical task of data ingestion while Azure managing the intricate process of data transformation. The data fabric stitches these diverse cloud environments into a cohesive, agile framework, enabling organizations to capitalize on each platform’s strengths while maintaining streamlined data management. By merging data governance, integration, and orchestration into a single architecture, this framework ensures that data flows seamlessly, enabling real-time insights and enhanced decision-making across the enterprise.
Cloud-Based Data Warehousing
In modern data management, data processing, storage, and analysis are entirely carried out in a cloud-based structure. The significant advantage of this approach is that it allows all business information to be stored and accessed in one place, streamlining data documentation and governance. Additionally, organizations don’t have to worry about the management, maintenance, and security of their data assets, as service providers like Azure, AWS, and GCP handle it. Businesses can benefit from the in-built security features (like role-based access control), data analytics tools, and APIs for their custom needs. The pay-as-you-go model further offers the flexibility to scale resources according to data volume, eliminating the need for heavy investment in on-premises infrastructure.
Take Airbnb as a prime example. To overcome the service administration challenges experienced by its original provider, the brand migrated to Amazon Web Services. Utilizing resources like Amazon RDS, EC2, Elastic MapReduce, and CloudWatch, Airbnb effortlessly manages big data, analyzes billions of customer events daily, and monitors all its server resources at 60% reduced operational costs.
Predictive Analytics
Knowing what data you have and extracting key insights from it can empower businesses to address issues proactively and gain a competitive edge. By analyzing historical data, AI models can predict future outcomes with remarkable accuracy, enabling companies to make informed, forward-looking decisions. Whether it’s forecasting sales, predicting customer behavior, or anticipating market shifts, AI-driven predictive analytics is transforming data into a strategic asset.
As Ken Corless, CTO of Cloud Consulting and Principal at Deloitte, explains, leveraging AIOps tools allows technology teams to continuously monitor a system’s behavior and performance in real time. These tools can sift through large volumes of data to identify anomalies, distinguishing between normal and potentially harmful events. Beyond just monitoring and alerting, AIOps empowers organizations to adapt their actions dynamically in response to current needs, events, or threats, making predictive analytics not only a tool for foresight but also a means for agile and responsive operations.
Areas Where AI Can Fail without Human Oversight
While artificial intelligence is a powerful resource for managing the data lifecycle, its implementation presents significant challenges that can hinder its effectiveness within organizations. Despite AI’s ability to enhance productivity and efficiency through process automation, it struggles to gain full confidence from stakeholders in these critical areas:
Data Quality & Reliability
As quoted by our expert, Mr. Rohit Bhateja “At the core of a trustworthy AI lies trustworthy data. The foundation of a consolidated, dependable AI enterprise lies in the data it ingests.” If the training data is unstructured, incomplete, or inaccurate, the outcomes AI/ML models will generate will be unreliable. AI systems can gather, process, and analyze millions of data points, but without proper training, this data holds little value. Accurately annotated data by subject matter experts is critical for AI model training and reliable outcomes.
Data Security
Data privacy and security in the age of AI have always been a debatable question. No matter how advanced the tool becomes, business owners hesitate to share their confidential and sensitive data on AL/ML tools as the risk of data breaches is prevalent. Using personal data in AI models without explicit consent carries significant risks, including potential legal consequences under data protection regulations such as GDPR and HIPAA. Unauthorized data usage can lead to privacy breaches, hefty fines, and harm to a brand’s reputation.
Human oversight in data governance is essential to ensure data is securely collected, processed, stored, and accessed. Those authorized to manage data platforms must establish and maintain strict protocols to protect information, both within and beyond the system.
Real-World Examples: How AI and Human Intelligence Together Drive Efficient Data Management
Humans are AI’s ethical compass, guiding it toward accurate and reliable outcomes. The synergy of subject matter experts and artificial intelligence can be powerful for businesses, enabling them to achieve enhanced operational efficiency, reduced overhead costs, and more time to focus on strategic initiatives.
Let’s explore how our human-in-the-loop approach helped enterprises improve the capabilities of their automated systems and data management processes.
Case Study 1: Provided Data Management Services for AI-Powered Brand Protection Platform
The client was a leading revenue recovery company with a proprietary AI-based platform that collects data from diverse web sources to help businesses identify copyright violations, brand impersonation, piracy, counterfeits, and distribution abuse. Although the AI tool was effective, it struggled to retrieve complete data from the web due to subpar training data quality. The client required clean, accurate, and well-structured data to optimize the performance of the brand protection tool. Additionally, the data scraped by the tool required enrichment and validation by subject matter experts.
Our web research experts and data professionals removed inconsistencies from the training data, appended the missing information in the scraped data, and validated the outcomes of the tool. As a result of our comprehensive data management support, the client saw a significant boost in the AI system’s accuracy and a 30% reduction in delivery timelines for their clientele.
Case Study 2: Implemented Centralized Data Lakes and BI Solutions for Effective Data Management and Analytics
The client was a renowned data management and consulting firm, assisting businesses in processing and handling large volumes of data (across diverse vertices like finance, healthcare, and retail). Their AI-driven platform transforms raw data into actionable insights. However, as the data came from disparate sources and diverse domains, the client struggled to process and maintain it.
They sought our expertise in data management and engineering to eliminate data silos and achieve a unified view of operations and systems. We streamlined their data management workflow through data cleaning, deduplication, and validation. Additionally, we evaluated their data points, existing technologies, and business requirements to design a scalable data lake architecture using AWS.
By implementing data ingestion pipelines and a business intelligence platform, we enabled real-time data processing, visualization, and reporting. Our approach not only improved the client’s ability to manage and analyze large datasets but also significantly reduced delivery timelines for the end users by 30%.
Redefine Data Management by Striking the Balance Between AI, Automation, and Human Oversight
Data is the new oil, but only useful when it is structured, accurate, complete, and updated. Mastering its management requires more than just advanced tools—it demands a strategic blend of AI, automation, and human oversight. With this synergy, you will not just merely process or manage data but unlock its true potential for strategic foresight and operational resilience. As a human-powered IT outsourcing firm, we blend our subject matter expertise with advanced data management tools to optimize client workflows while maintaining strict compliance with regulations like GDPR and HIPAA.